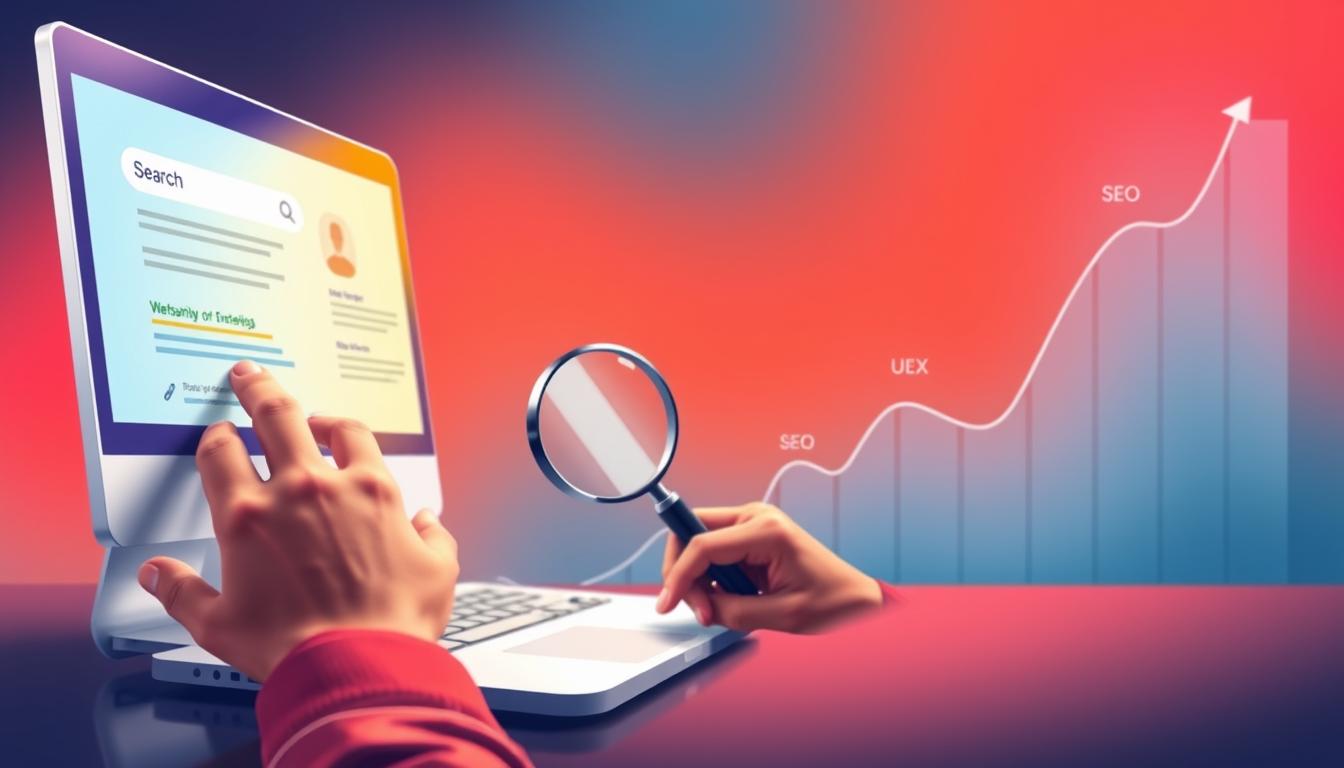
Leverage AI to Boost Cybersecurity Defenses
Cybersecurity threats grow more complex each year, overwhelming traditional defenses. AI cybersecurity technology now offers a critical advantage, enabling organizations to detect and respond to attacks faster than ever. From phishing to ransomware, AI adapts to evolving risks in real time.
Leading companies like IBM and Darktrace use ai in cybersecurity solutions to reduce breach detection times by up to 60%. These systems analyze vast data streams, identifying anomalies human teams miss. Machine learning models continuously improve, creating proactive shields against cybercriminals.
This article explores how AI transforms security strategies, from threat detection to automated responses. Readers will learn practical steps to integrate AI tools like AI-powered firewalls and behavioral analytics into their infrastructure. The coming sections reveal how these innovations are reshaping digital protection for the modern era.
Understanding AI in Cybersecurity Solutions
Artificial intelligence (AI) is reshaping how organizations defend against cyber threats. To unlock its potential, it’s critical to grasp its foundational concepts and applications in security systems.
What is AI?
AI refers to systems capable of performing tasks requiring human-like decision-making. In cybersecurity, ai in cybersecurity solutions focuses on narrow AI, which specializes in specific functions like threat detection rather than general problem-solving. Unlike human cognition, these systems analyze vast datasets to identify anomalies.
The Role of Machine Learning
Machine learning for cyber defense powers adaptive security by enabling systems to improve without explicit instructions. Three core approaches drive this process:
- Supervised learning: Uses labeled data to recognize known attack patterns (e.g., phishing emails).
- Unsupervised learning: Discovers hidden threats by clustering unlabeled data to spot irregularities.
- Reinforcement learning: Adjusts responses in real time based on feedback from network behaviors.
Key Technologies in Use
Modern ai in cybersecurity solutions integrate advanced technologies such as:
- Natural Language Processing (NLP): Analyzes text in threat intelligence reports to detect linguistic markers of phishing scams.
- Deep Learning Neural Networks: Mimic human brain structures to classify malware with high accuracy.
- Computer Vision: Scans network visuals (e.g., traffic graphs) to flag suspicious activities.
These technologies work together to create proactive defense layers, reducing human error and response times.
Benefits of AI in Cybersecurity
AI transforms cybersecurity through three core advantages: faster threat identification, immediate incident containment, and streamlined workflows. These capabilities address the growing complexity of cyber threats while reducing risks to critical systems.
Enhanced Threat Detection
Advanced threat detection AI identifies hidden risks by analyzing network traffic and user behavior. Companies like Darktrace use neural networks to spot zero-day exploits and fileless attacks. A 2023 Gartner study reveals organizations with such systems reduced breach incidents by 40% compared to traditional methods.
Real-time Response Capabilities
“AI-powered cybersecurity platforms reduce response time from days to seconds,”
states Forrester Research. Tools like Palo Alto Networks’ Cortex XDR automatically isolate threats as they emerge. This real-time action slashes the average cost of a data breach by $1.2 million, according to IBM’s 2023 Cost of a Data Breach Report.
Operational Efficiency
AI reduces manual workload by automating routine tasks. CrowdStrike’s Falcon platform cuts false positives by 60%, letting teams focus on strategic planning instead of sifting through alerts. Automation of patch management and vulnerability scans saves 200+ hours annually for teams at Fortune 500 firms like JPMorgan Chase.
AI-Driven Threat Intelligence
Modern cybersecurity strategies increasingly depend on using AI in threat intelligence to uncover hidden attack patterns. By integrating AI-driven cybersecurity solutions, organizations can now process vast data streams to preempt cyber threats before they strike. This shift from reactive to proactive defense hinges on three core processes: data gathering, pattern identification, and predictive forecasting.
Data Collection Techniques
Effective threat intelligence starts with data aggregation. AI systems gather information from:
- Dark web forums and underground marketplaces
- Open-source social media and hacker blogs
- Network traffic logs and endpoint behavior metrics
- Historical breach databases
Analyzing Threat Patterns
Machine learning algorithms detect hidden connections between seemingly unrelated events. Clustering techniques group similar attack vectors, while anomaly detection flags irregular activity. Behavioral analysis tools identify phishing campaigns targeting specific industries, as seen in 2023 ransomware patterns affecting healthcare networks.
Predictive Analytics for Future Threats
“Predictive models now forecast 60% of advanced threats 48 hours before execution,” says a 2023 study by MITRE. These systems simulate attack scenarios to prioritize patching vulnerabilities in critical systems first. By modeling threat actor behaviors, AI reduces the average dwell time of undetected breaches from 280 days to under 72 hours.
These advancements in AI-driven cybersecurity solutions empower teams to act before threats materialize, turning historical data into actionable defense strategies.
Automating Security Measures with AI
Cybersecurity automation with AI is reshaping how organizations defend against threats. AI-powered cybersecurity platforms now enable systems to act autonomously, cutting response times and minimizing human error. These tools adapt to evolving risks while maintaining compliance and operational continuity.
Automated Incident Response
Automated systems trigger actions like isolatinging compromised devices or blocking malicious IP addresses automatically. For instance, Darktrace’s AI analyzes events in real-time, executing remediation steps without human input. Metrics show this reduces mean time to respond (MTTR) by up to 35%, according to a 2023 SANS Institute report.
AI-Powereded Firewalls
Modern firewalls use machine learning to assess traffic dynamically. Instead of static rules, solutions like Palo Alto Networks’ Next-Generation Firewall (NGFW) evaluate user behavior and context. This adaptive approach blocks zero-day attacks and phishing attempts before they breach networks.
User Behavior Analytics
AI models create baselines for normal activity, flagging deviations instantly. Okta’s Identity Engine detects unusual login patterns, such as a user accessing restricted data at odd hours. This prevents insider threats and credential theft before damage occurs.
“AI-driven automation turns reactive defenses into proactive barriers.” — MITRE Corporation, 2023 Threat Defense Report
Deploying these systems requires integratinging AI tools with existing infrastructure. Companies like Bank of America cut breach detection times by 60% after adopting AI-powered automation. Prioritizing scalability and interoperability ensures seamless upgrades without disrupting daily operations.
AI and Malware Detection
Modern AI algorithms for protecting against cyber attacks are reshaping how organizations combat malware. Traditional signature-based methods often fail to detect novel threats, but artificial intelligence security software now analyzes behavior and patterns to identify risks proactively.
Identifying Malicious Activity
Advanced systems leverage machine learning to assess code behavior, file anomalies, and execution sequences. Key methods include:
- Code analysis to flag hidden malicious functions
- Dynamic sandboxing to observe runtime behavior
- Pattern recognition for abnormal network connections
Using AI for Heuristic Analysis
Artificial intelligence security software employs heuristic analysis to uncover threats without prior threat signatures. Techniques include:
- Code emulation to test file behavior in isolated environments
- Behavioral scoring systems that flag suspicious actions
- Adaptive models that refine detection over time
Case Studies of Successful Detection
“Our AI platform identified a polymorphic banking Trojan that evaded 98% of traditional tools through behavioral analysis alone.” – 2023 Cisco Threat Report
In 2022, Palo Alto Networks used AI algorithms to detect a fileless malware campaign targeting healthcare providers. The system identified memory-based attacks by analyzing process anomalies, preventing data exfiltration from 15 hospitals. Another case involved Microsoft’s Defender detecting ransomware variants by tracking unusual encryption patterns, reducing response times by 60%.
The Impact of AI on Risk Management
AI-driven cybersecurity solutions are redefining risk management by shifting from manual audits to continuous, adaptive evaluations. These tools provide real-time insights into an organization’s security posture, addressing vulnerabilities before they become crises.
Analyzing Vulnerabilities
Traditional vulnerability scans are limited to scheduled checks. AI-driven systems, however, monitor networks and applications 24/7. They flag weaknesses, rank them by severity and business impact, and track fixes until resolved. For instance, AI identifies outdated software in critical systems, prioritizing patches to prevent breaches.
Risk Assessment Automation
AI cybersecurity technology replaces manual risk scoring with machine learning-driven analysis. Algorithms assess thousands of variables simultaneously, including:
- Threat likelihood and historical breach data
- Asset value and operational impact
- Compliance alignment and control effectiveness
Quantitative scores replace subjective judgments, reducing assessment time by up to 50% while improving accuracy. Organizations now quantify risks with metrics like “risk exposure scores,” enabling faster decision-making.
Tailored Security Solutions
AI-driven cybersecurity solutions deliver customized defenses instead of one-size-fits-all strategies. A retail company, for example, might prioritize protecting payment systems over less critical assets. This precision reduces wasted resources while boosting protection. Metrics like a 35% drop in incident response time and a 20% ROI increase show measurable benefits.
Successful implementation demands quality data and alignment with existing governance frameworks. Organizations must ensure data accuracy and compatibility with tools like SIEM platforms to fully harness AI’s capabilities.
Integration of AI with Existing Security Tools
Modern organizations rely on legacy security systems, but artificial intelligence security software is bridging gaps between old and new. By embedding AI into existing frameworks, businesses unlock smarter threat detection without overhauling infrastructure.
Enhancing SIEM Systems
Traditional Security Information and Event Management (SIEM) platforms face limitations in handling modern threats. AI transforms these systems by:
- Reducing false positives through pattern recognition
- Contextualizing alerts using historical data
- Identifying multi-stage attack chains
IBM QRadar and Splunk Phantom now offer AI modules that analyze event logs in real time, cutting investigation time by up to 60% according to 2023 Gartner reports.
Combining AI with the Cloud
Cloud-native AI-powered cybersecurity platforms like AWS GuardDuty and Microsoft Azure Sentinel enable:
- Scalable threat hunting across global networks
- Shared threat intelligence databases
- Automated policy updates without on-premise hardware
Organizations using cloud-based AI solutions report 45% faster incident containment, but must address encryption requirements for cross-cloud data transfers.
Interoperability Benefits
AI acts as a unifying layer between tools like CrowdStrike Falcon and Palo Alto Networks through:
- API-driven data exchange
- Standardized threat format sharing (STIX/TAXII)
- Automated workflow orchestration
A 2024 SANS Institute study found integrated systems reduce manual coordination by 35%, though requires 15-20% more computational resources initially.
“Interoperable AI systems turn fragmented security tools into cohesive defense networks,” stated Dr. Emily Chen, CTO of Darktrace, during RSA Conference 2024. “This synergy is critical for defending against multi-vector attacks.”
Challenges Facing AI in Cybersecurity
While ai in cybersecurity solutions promises advanced protection, real-world adoption encounters hurdles demanding careful navigation. These challenges range from regulatory barriers to technical limitations that require strategic planning to overcome.
Data Privacy Concerns
Implementing machine learning for cyber defense often requires handling sensitive data, creating tension with privacy laws like GDPR and CCPA. Organizations must balance training model accuracy with compliance. Techniques like federated learning—where data remains decentralized—offer a path forward. Anonymization and differential privacy methods reduce exposure while maintaining utility. Legal teams must collaborate with engineers to ensure frameworks like these align with regulatory standards.
Dependence on Quality Data
AI systems rely on robust, unbiased datasets to function effectively. Challenges arise when rare attack types lack sufficient training examples. Poor-quality data introduces biases, skewing threat detection. Solutions include:
- Synthetic data generation to simulate attack scenarios
- Data augmentation to diversify training inputs
- Regular audits to identify and correct dataset biases
Limitations of AI Algorithms
Current AI tools remain vulnerable to adversarial attacks designed to deceive detection systems. Explainability issues also persist, as complex models often operate as “black boxes,” complicating trust. A
NIST 2023 report noted 60% of enterprises still require human analysts to validate AI findings
. Hybrid approaches combiningmachine learning for cyber defensewith human expertise remain critical to address these gaps.
Future Trends in AI Cybersecurity Solutions
As cybersecurity evolves, AI continues to redefine boundaries. Three key trends will dominate the next decade: quantum computing integration, advanced deep learning models, and symbiotic human-AI workflows.
AI and Quantum Computing will reshape encryption landscapes. While quantum systems could crack today’s algorithms, companies like IBM and Google are already developing AI algorithms for protecting against cyber attacks that resist quantum decryption. Research shows quantum-resistant cryptographic standards (e.g., NIST’s post-quantum cryptography) could be standardized by 2026, enabling AI to dynamically adapt encryption protocols in real time.
The Rise of Deep Learning brings breakthroughs in pattern recognition. Transformers and graph neural networks now process unstructured data like network traffic to identify hidden attack vectors. For example:
- Graph neural networks map attack paths across enterprise systems
- Self-supervised learning reduces reliance on labeled data for advanced threat detection AI
- Microsoft’s DeepSec framework reduced zero-day detection time by 40% in 2023 trials
Human-AI Collaboration emerges as the new standard. A 2024 MIT study found that hybrid teams cut incident resolution times by 35% through:
- AI handling anomaly scoring and initial triage
- Analysts focusing on strategic decisions
- Continuous feedback loops improving model accuracy
“The future lies in systems where humans set the guardrails while AI operates within those boundaries,” said Dr. Elena Torres, CTO of CyberSentinel Labs.
Organizations should start pilot programs with AI-augmented threat hunting tools and invest in explainable AI systems to maintain transparency. These trends position AI not just as a tool, but as an integral partner in evolving cybersecurity postures.
Building a Cybersecurity Strategy with AI
Adopting AI requires a tailored plan that aligns with business priorities. Follow a three-step approach to integrate cybersecurity automation with AI effectively without disrupting operations. Start by identifying where AI adds maximum value and scale strategically.
- Assess Needs First
Audit existing systems using frameworks like NIST to pinpoint gaps. Map risks to AI applications such as automated incident response or using AI in threat intelligence to prioritize high-impact areas. Benchmark against industry standards to avoid over-investing in low-risk domains. - Test Before Scaling
Begin with small-scale deployments like AI-powered email filtering tools from vendors like Proofpoint or CrowdStrike. Monitor pilot outcomes before expanding. Training programs for IT teams reduce resistance to change and ensure smooth adoption. - Track Results Continuously
Use metrics like reduced dwell time for breaches and improved threat detection rates. Adjust strategies based on feedback loops. Budget for ongoing updates to AI models as threat landscapes evolve.
Real-world examples show companies like JPMorgan Chase cut breach response times by 60% through phased AI adoption. Regular reviews of KPIs like compliance audit scores ensure long-term success.
Case Studies of Successful AI Deployments
Real-world implementations of AI-driven cybersecurity solutions reveal how organizations across industries are redefining security postures. These examples highlight actionable insights for adopting AI in cybersecurity solutions without repeating earlier technical details.
Fortune 500 Companies
Leading enterprises like JPMorgan Chase deployed AI to reduce fraud detection time by 70% using machine learning models. Mayo Clinic integrated AI systems to safeguard electronic health records, detecting 30% more breaches than traditional methods. Siemens optimized operational technology security through AI, preventing supply chain disruptions in manufacturing sectors.
Startups Innovating in Security
Emerging firms such as Darktrace and CrowdStrike lead breakthroughs. Darktrace’s AI autonomously disrupts cyberattacks in real time, while CrowdStrike Falcon uses behavior analysis to block ransomware before execution. These startups demonstrate how agile AI applications protect cloud and endpoint environments without relying on predefined signatures.
Lessons from Implementation Challenges
Not all deployments succeed. One retail giant’s AI model failed after training on biased datasets, mislabeling normal activity as threats. Another financial institution underestimated integration costs, delaying project timelines. These missteps underscore the need for rigorous data curation and phased implementation strategies.
Organizations adopting AI must balance innovation with practical planning. Case studies prove that aligning AI capabilities with specific business needs—and learning from past errors—ensures sustainable cybersecurity improvements.